The two questions HR should be asking about AI
Our Q&A with VP Amber Foucault on key questions to ask about AI and how to know when a product is developed to meet your HR team’s needs.
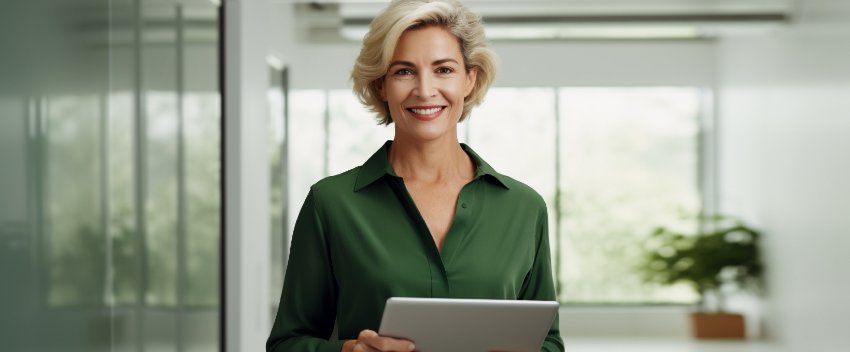
Human capital management (HCM) innovation is changing the way organisations operate through automation, talent intelligence, and machine learning designed to help solve complex business problems. Companies are racing to incorporate artificial intelligence (AI) into their systems, but not all solutions are created with HR needs in mind. There are often mixed messages on how AI-based tech can actually make an impact for HR leaders and their functional areas to drive value for the business.
More leaders are motivated to add AI technologies to their tech stack, but they are walking a careful line between embracing new technology that could give them a competitive edge and managing the risk that these new innovations could bring. The organisations that stay ahead of the pack will embrace AI, automation, and self-service technologies that help simplify and streamline repetitive tasks to refocus their workforces on the most important and impactful work.
At the Women in Tech Global Conference in April 2024, Amber Foucault, Vice President of Product Applications spoke on integrating AI and machine learning (ML) into the product development process. Below are some of her key takeaways for HR leaders looking into AI:
What are some of the biggest challenges you see for developing AI-enhanced products?
The way I see it is with two questions. Have you selected a problem that AI should solve? Because people can get really excited about using AI, but they may not reflect on exactly what people are looking to solve with AI.
I don't know if product teams are always selecting the best problems that need to be solved with AI. What is the benefit to a payroll administrator or an HR business partner? A good example of the right type of question to solve for a payroll administrator might be, “How can AI deliver suggestions to help me understand what's causing anomalies in my payroll without me having to ask?” Building those types of intelligent nudges right into the system is a product innovation that aligns with actual needs. This is a good problem for AI to solve.
And this leads to the second question, which is: What level of accuracy do you need to solve this problem? Another key area of alignment with real business needs is helping with payroll accuracy. Let’s say I'm augmenting a payroll administrator’s job with tasks completed with AI. I must strive to be 100% accurate because this task is responsible for paying someone accurately. Do I have the data and ability to be 100% accurate with help from AI? Because that's the only acceptable outcome on the other side of payroll.
Or consider the challenge of skills matching for talent. Could I afford to be 87% accurate? In terms of skills matching, there could be grey lines between what one skill involves versus what another one involves. In that case we’re looking at a different challenge than we were with payroll, where avoiding over- or under-paying someone is non-negotiable.
So, identifying clean and clear defining problem sets and understanding how accurate you need to be are two important questions that need to be asked.
How has the process for building new products changed with the emergence of AI and ML technologies?
I think a big misconception is that developing products with AI and ML is the same as building other product processes. The cost of failure is significantly less for building a mobile app than building an AI product because you must be able to continually invest in an AI model to drive accuracy. For example, if I wanted a consumer to purchase a pair of sneakers off a new app, I could try 20 different versions of that user flow and put 20 different options in front of a testing group of 100 people to test which versions work. That’s a lower cost for me to do compared to the intense processes of evaluating an AI model with expensive data sets, the suite of tools that you need to use alongside testing, and the consistent refining and investing.
The evaluation stage is an important part of training an AI model so that you might understand its accuracy and behaviors. It takes a lot of time, tooling, and effort to invest in proper evaluations, and this is in addition to other aspects of the product build process. With the mobile app example, maybe you could figure out a way to do that within two months. However, depending on your AI model, you're most likely looking at substantially longer timelines.
Another substantial hurdle is the cost of scaling. You may have the perfect problem, and enough information to train and evaluate an AI model, but how can you scale that to customers at the right cost? We are watching certain AI companies struggle to balance the scaling costs of their products against their drive for continuous innovation.
Some people are hesitant about incorporating AI into their HR processes. What does trustworthy innovation look like in practice?
When we talk about ethical AI and what trustworthy innovation means for us, we think about an AI-enhanced approach in three ways. We separate it into a single data model, strict data governance, and secure data isolation. And that leads us to a single source of truth for people data.
It's very people-centric, and it's making sure that we understand how we protect that people data. That’s the most important thing that we can focus on.
But I will go back to this idea about how accurate do I have to be? Because this is where ethical conversations need to start. As an example, if we were building autonomous driving cars, we would have to say that the cost of failure is human life. That's a very high cost.
So, with that example in mind, how accurate does your model have to be? In the example of autonomous cars, when you’re 99% accurate, that’s still one out of 100 lives you are jeopardising. That’s a very high cost of failure. What will you be willing to accept?
What should companies consider when evaluating AI technology for their HR tech stack?
I was just at a payroll workshop where out of 250 attendees, only 7% of those surveyed said they were comfortable understanding new technology like AI. It’s my job to help demystify what AI is meant to do inside of a product. Some people may be thinking: "Is AI going to steal my job? How is this going to affect me, and how am I supposed to trust this?” It can be hard to conceptualise because human nature is for us to fear what we don’t know.
The power of AI stems from efficiency and automation, so step one in my thought process is evaluating if I can help automate tasks for our customers. What repetitive tasks do you face continuously that are prone to human error? Can those be mapped back in an intelligent way to an AI-enhanced solution that will help free up your time so you can focus on more important things?
At Dayforce, we believe that AI must be part of the foundation of our product. , It should be easy to use and easy to incorporate into our customers’ everyday lives. Approaching AI like a bolt-on to your product suite isolates growth potential and expansion of AI capabilities. However, when it's one of the foundations of your product, it opens worlds of possibilities, allowing you to think about automation. For example, AI can allow you to identify payroll anomalies and help you resolve them quickly and easily – speeding up your pay run cycle.
In the end, maybe only 7% of surveyed payroll professionals are comfortable talking about AI solutions, but more didn't even recognise they were using technology enhanced by AI. Those are the kind of little leaps and bounds you want to be able to take towards building adoption and clarity within your organisation.
You may also like:
Ready to get started?
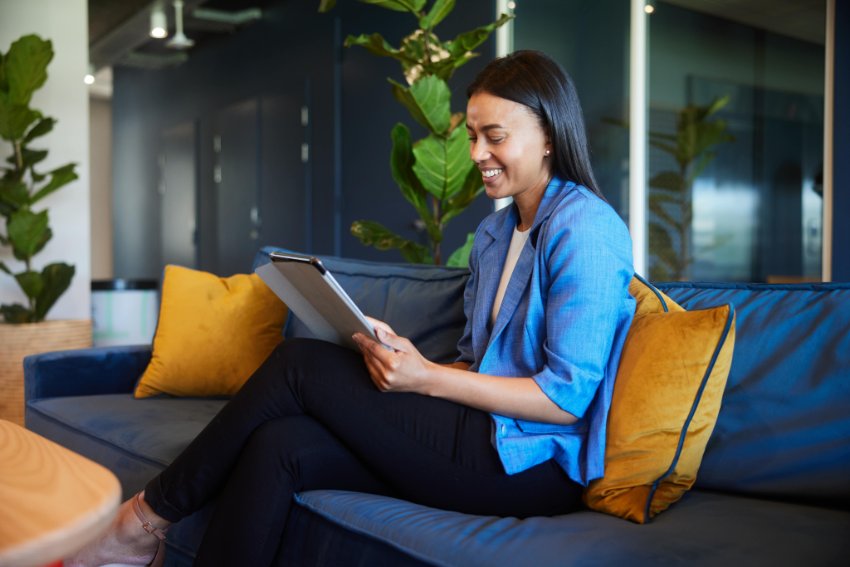